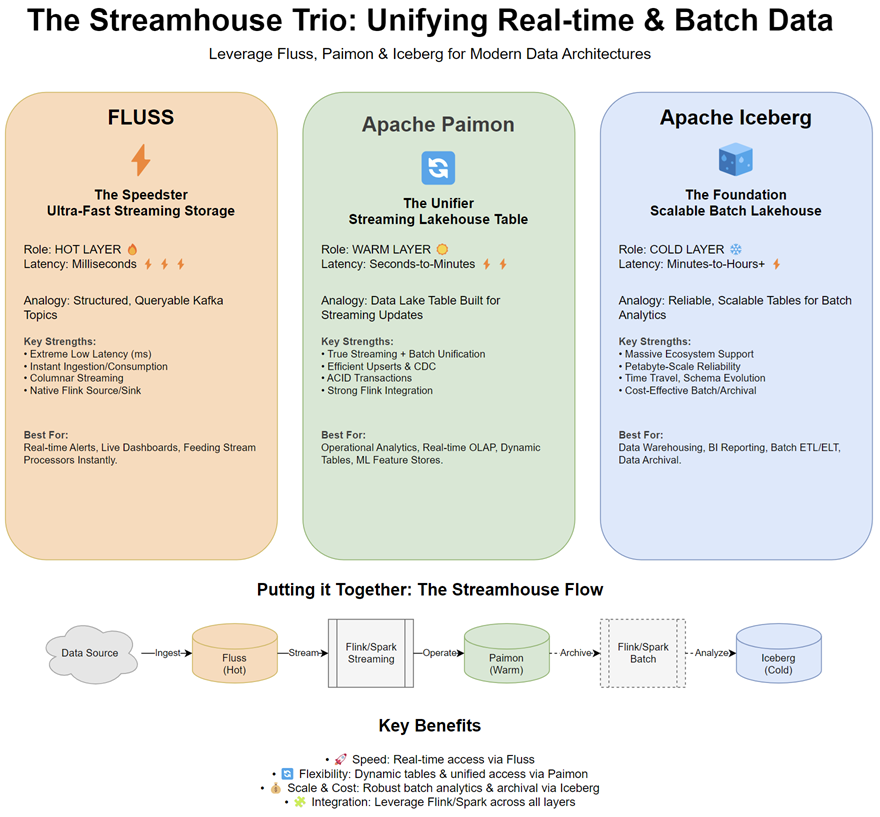
The world of data is converging. The traditional divide between batch processing for historical analytics and stream processing for real-time insights is becoming increasingly blurry. Businesses demand architectures that handle both seamlessly. Enter the “Streamhouse” - an evolution of the Lakehouse concept, designed with streaming as a first-class citizen.
Today, we’ll introduce three key open-source technologies shaping this space: Apache Paimon™, Fluss, and Apache Iceberg. While each has unique strengths, their true power lies in how they can be integrated to build robust, flexible, and performant data platforms.