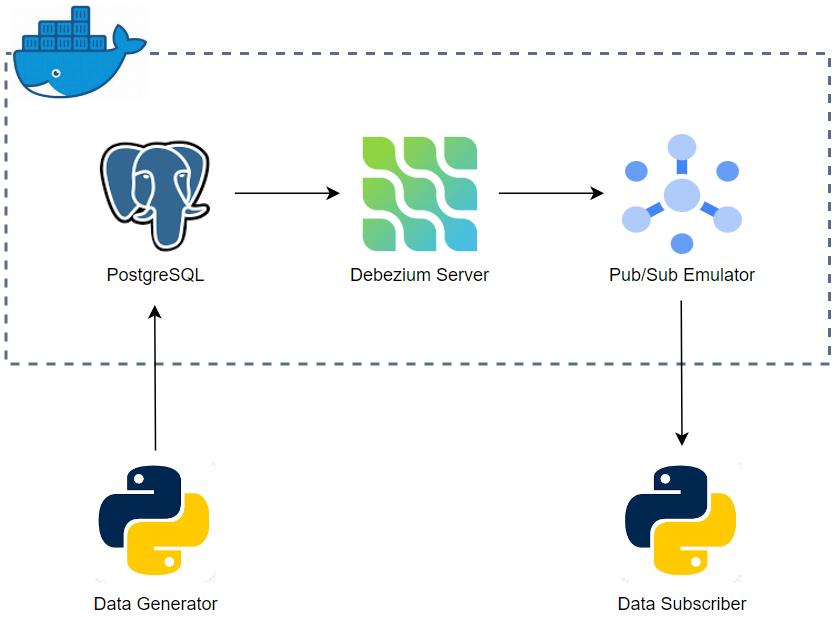
Change data capture (CDC) is a data integration pattern to track changes in a database so that actions can be taken using the changed data. Debezium is probably the most popular open source platform for CDC. Originally providing Kafka source connectors, it also supports a ready-to-use application called Debezium server. The standalone application can be used to stream change events to other messaging infrastructure such as Google Cloud Pub/Sub, Amazon Kinesis and Apache Pulsar. In this post, we develop a CDC solution locally using Docker. The source of the theLook eCommerce is modified to generate data continuously, and the data is inserted into multiple tables of a PostgreSQL database. Among those tables, two of them are tracked by the Debezium server, and it pushes row-level changes of those tables into Pub/Sub topics on the Pub/Sub emulator. Finally, messages of the topics are read by a Python application.