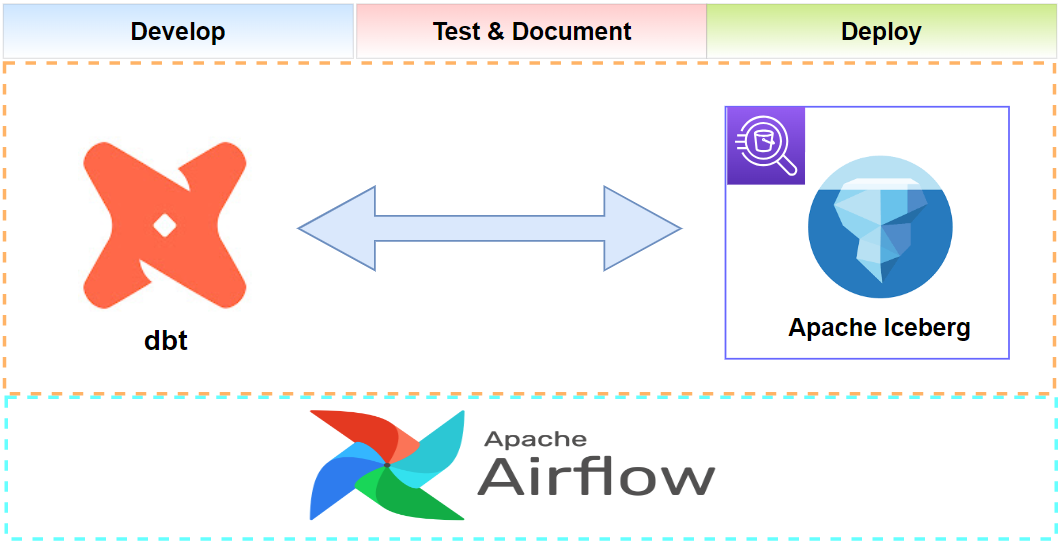
In Part 5, we developed a dbt project that that targets Apache Iceberg where transformations are performed on Amazon Athena. Two dimension tables that keep product and user records are created as Type 2 slowly changing dimension (SCD Type 2) tables, and one transactional fact table is built to keep pizza orders. To improve query performance, the fact table is denormalized to pre-join records from the dimension tables using the array and struct data types. In this post, we discuss how to set up an ETL process on the project using Apache Airflow.