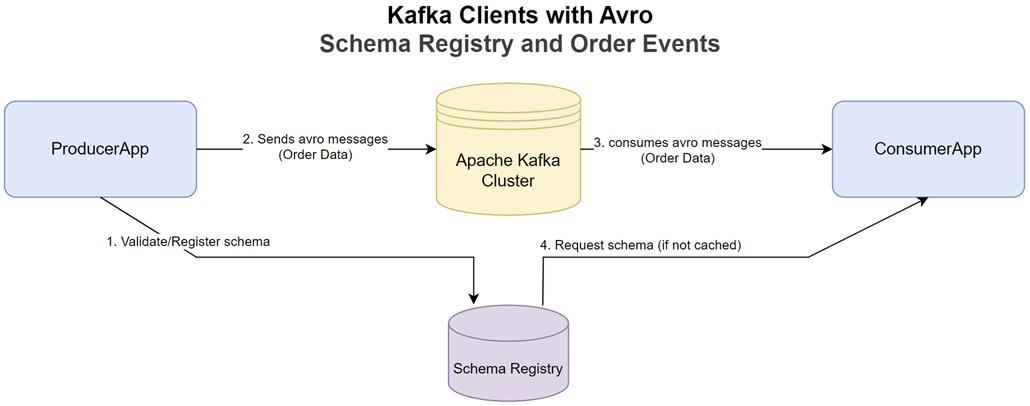
In this post, we’ll explore a practical example of building Kafka client applications using Kotlin, Apache Avro for data serialization, and Gradle for build management. We’ll walk through the setup of a Kafka producer that generates mock order data and a consumer that processes these orders. This example highlights best practices such as schema management with Avro, robust error handling, and graceful shutdown, providing a solid foundation for your own Kafka-based projects. We’ll dive into the build configuration, the Avro schema definition, utility functions for Kafka administration, and the core logic of both the producer and consumer applications.