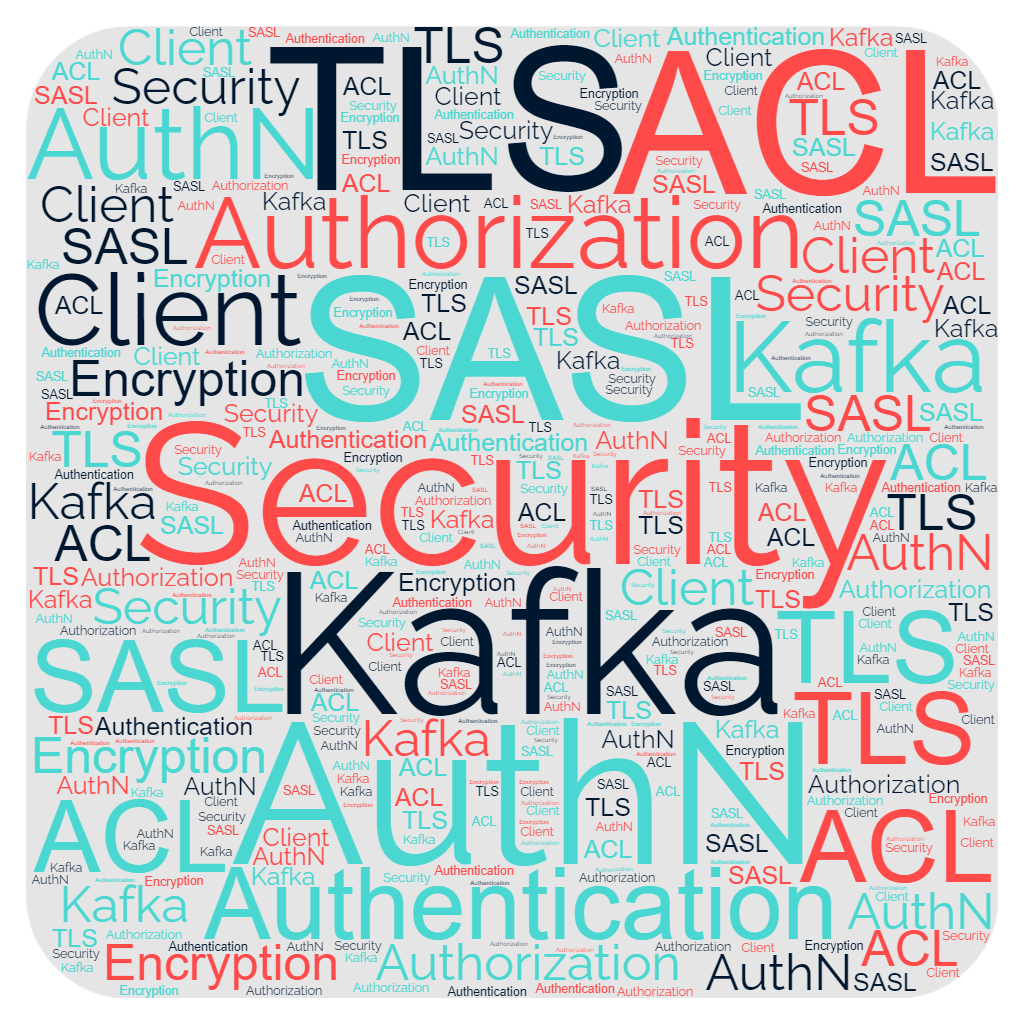
In the previous post, we discussed TLS (SSL or TLS/SSL) authentication to improve security. It enforces two-way verification where a client certificate is verified by Kafka brokers. Client authentication can also be enabled by Simple Authentication and Security Layer (SASL), and we will discuss how to implement SASL authentication with Java and Python client examples in this post.