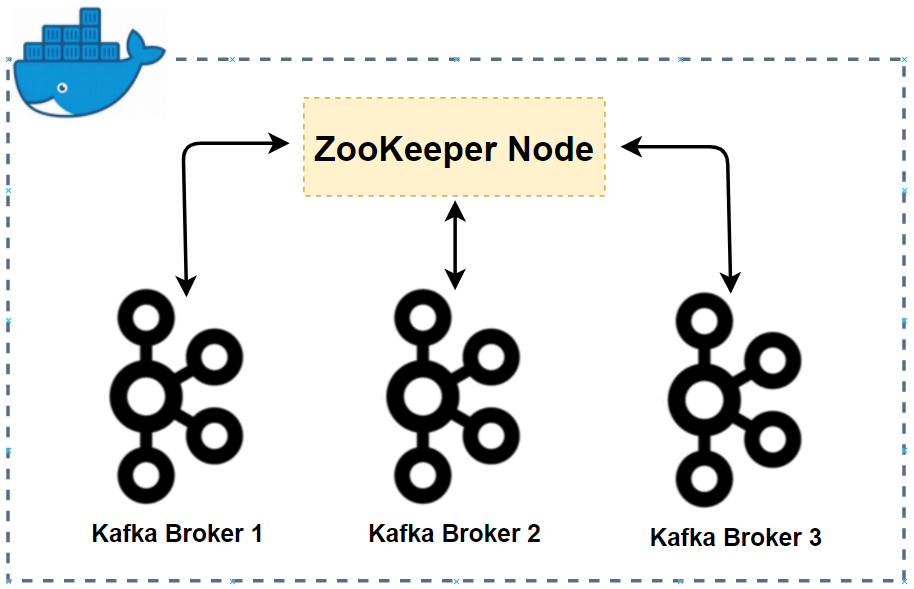
Apache Kafka is one of the key technologies for modern data streaming architectures on AWS. Developing and testing Kafka-related applications can be easier using Docker and Docker Compose. In this series of posts, I will demonstrate reference implementations of those applications in Dockerized environments.