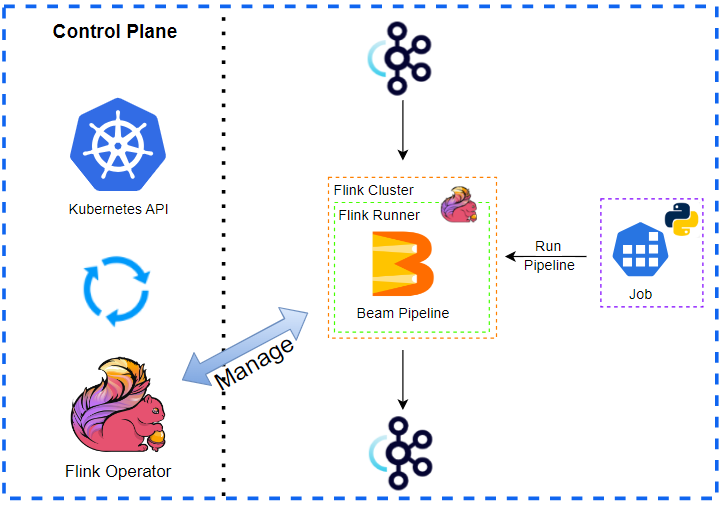
In this post, we develop an Apache Beam pipeline using the Python SDK and deploy it on an Apache Flink cluster via the Apache Flink Runner. Same as Part I, we deploy a Kafka cluster using the Strimzi Operator on a minikube cluster as the pipeline uses Apache Kafka topics for its data source and sink. Then, we develop the pipeline as a Python package and add the package to a custom Docker image so that Python user code can be executed externally. For deployment, we create a Flink session cluster via the Flink Kubernetes Operator, and deploy the pipeline using a Kubernetes job. Finally, we check the output of the application by sending messages to the input Kafka topic using a Python producer application.